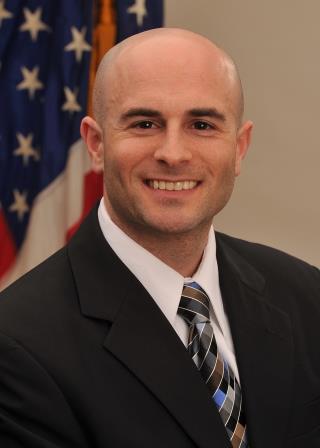
Steven Posnack serves as director of the Office of Standards and Technology. In this role, Mr. Posnack advises the national coordinator, leads the ONC Health IT Certification Program, and directs ONC’s standards and technology investments through the ONC Tech Lab, which organizes its work into four focus areas: pilots, standards coordination, testing and utilities, and innovation. He led the creation of the Interoperability Standards Advisory, the redesign of ONC’s Certified Health IT Product List (CHPL), created the Interoperability Proving Ground, and developed the C-CDA Scorecard. In June, Mr. Posnack’s office issued a Patient Matching Algorithm Challenge. PARCA eNews spoke with him about patient matching and challenges of ensuring accurate identification of all parts of a patient record.
How did we get to where we are with patient matching?
The point of origin would be HIPAA the law of 1996 and that included within it a number of administrative simplification requirements, including different identifiers for healthcare providers, for health insurance plans, and there was one related to identification of patients.
In subsequent fiscal years Congress passed a rider or provision for lack of a better word to an appropriations bill that prohibited HHS (Health and Human Services) from using funding to go through a regulatory process to adopt a standard patient identifier. That was before my office (ONC) was even formed, because we were created in 2004.
ONC was created under the Bush administration via Executive Order and interoperability and health IT adoption were foremost on the minds of leaders at that time. We began trying to solve this problem of identifying patients within and across various systems. As soon as it was clear that a unique patient ID at a national level was not going to happen and with the widespread adoption of electronic health records, people became more focused on how do we match patients across these different systems and use computers for what they are good at.
My office specifically got involved through a project that I was the project officer on, the Health Information Security and Privacy Collaboration, which started in 2006. We were looking at variations in state privacy and security laws and other impediments to health information exchange that we needed to be aware of and where we (ONC) could help at a state level or help with federal policy, and patient matching was certainly one of those impediments.
In early 2009, we co-authored a paper with Shaun Grannis (MD), an expert in patient matching at the Regenstrief Institute, Inc., where we looked for certain commonalities people were using to do matching, the relative value of certain data elements, and things of that nature. Since then we've done other research in the form of exploratory papers that have involved some of our federal advisory committees’ opinions and recommendations as well as landscape analyses to better understand the state of the market and how healthcare providers and other stakeholders are approaching patient matching.
In 2014, which was the last time we did a full scan, we published a patient matching report. In terms of the present tense, we've been working in other areas where patient matching and aggregation and linking of data are important for precision medicine, clinical trials research, population health analytics and other types of big data-related projects – contexts in which you are looking to aggregate patient data. So those are some of the other industry use cases or drivers that are of interest to us. What we've heard from stakeholders is that they are continuing to be interested in receiving guidance.
Would you say that patient matching is the key to interoperability?
It definitely one of the critical infrastructure components that we have today and that everyone is striving to get right. There are a few other dimensions to make interoperability happen but this is definitely one of the key components.
What are a couple of the most common approaches to developing algorithms to do this?
There are two kinds of buckets that you can group the different patient matching approaches into, one is called probabilistic and the other is deterministic. There is also a hybrid between the two. There are usually layers where the algorithms will be deployed that result in a certain level of assurance of match rates, and often we see some that can't be matched or are fuzzy and they'll be put into a kind of secondary holding pen where a human will have to do some additional adjudication. Overall, the idea is to minimize that as much as possible so you don't have hundreds of people working on matching issues. On a general level those are the two approaches people start with. Most of the time now we are seeing probabilistic approaches.
There are other things you can do beyond the specific algorithm choice that folks work on in terms of tuning; such as, depending on your use case, you could set a very low tolerance for false positives. So the algorithm might be tweaked to reduce the number of false positive and that will be something that has trade-offs in terms of how the matching performs. You may have others where you have a higher tolerance of false positive in instances where you don't have clinical treatment in mind, but say pairing claims together for population based research. Depending on the type of work or analyses you are doing you may be able to tolerate a certain level of false positives, or on the flip side, where you want to reduce the number of false negatives.
What about voluntary universal healthcare identifier, is that one of the approaches you were talking about?
That is a private sector industry effort that has existed for quite a while now and it is certainly an approach that organizations can take if they wanted to voluntarily group together and engineer a common architecture and approach to using unique IDs. Another you might have seen is the CHIME patient identity challenge which has a $1 million prize. This X-prize challenge is complementary to the challenge we put out. They (CHIME) are really looking at the future for breakthroughs beyond the current matching algorithms that we have. Our challenge is aimed at understanding where the current state of matching is today and looking for the best of the current algorithms.
The approach we're taking with the challenge is to get a better industry-wide definition in terms of ‘here are the benchmarks we think are important in the current state,’ and to get greater visibility around how current algorithms are performing on those key benchmarks.
There is a statistical term used for overall accuracy called F-score along with “precision” and “recall,” which are additional statistical terms in this space. As part of the challenge we want to see what the current state is in terms of what the benchmarks are industry-wide for performance within both commercial and open source algorithms and products that are out there.
Equally, we wanted to try to track other industries outside of healthcare. I'm sure you've flown, and anytime you go to that airport kiosk and swipe your credit card and it says it can't find you, you have to type in some additional information. The airlines all have record locator and flier identification algorithms that they are using to identify you. It is a little easier compared to healthcare where the transmission might not be known at the time it happened. There are many other industries where people have experience in this type of work and we are hoping that they may be attracted to participate in the challenge as well.
Are you trying to increase transparency between vendors with proprietary algorithms?
Yes, we hope that’s one outcome of the challenge. Algorithm performance is a bit of a black box in terms of industry visibility, which we hope to get a bit more insight into through the challenge.
The difficulty we face with patient matching is that it is affected by the quality of the data used to do the matching, like the saying ‘garbage in garbage out.’ As you and I interact with the healthcare system there are processes that collect a lot of data about us and a lot of opportunities for error with transpositions, and other types of entry issues. These make it harder for the patient matching algorithms to work the way they are designed. In addition, you also have cultural sensitivities in certain cultures, like not giving birthdays, and multiple names that are not in traditional Anglo Saxon formats, and other data variabilities that make the work challenging and extract creativity out of engineers.
The HIT Standards Committee has set a goal of 99% accuracy for patient matching, how far are we from reaching that goal?
That's a good question and is one we don't have a lot of data for. The other point you could look at is nationwide Interoperability Roadmap that ONC published in 2015. We have a subsection around individual data matching and for the end of 2017 we had set a goal for industry to reduce internal duplicate records percentage to 2 percent.
It is a multi-dimensional problem that you can look at from different angles like a Rubik’s Cube that you can focus on one face of the cube for different types of things. Articulated in the Roadmap was internal to an organization. For example, if I show up today and they record my information and then show up three weeks from now, we want to make sure that doesn't result in a completely new record entry for me.
Not only is there an issue of matching records across organizations as my information is transported from point A to point B, but it is also interactions within a single healthcare system depending on who is doing the intake. If there are errors, once you get past a particular threshold, and I don't know how some of these are built, but it does create a new record. For example if they spelled my name with a “ph” instead of “v” it can create a cascading effect such that the system may not treat me as the original Steve that came in three weeks ago. So that is an underlying theme related to matching and eliminating duplicate records.
My mom had an incident, and this was on a paper system, someone had the same name and same birthday and so their records got merged and when she was at the doctor's office after a few questions, it became obvious they had put someone else's chart into her record. People look at that and the dichotomy between paper and electronic environment becomes apparent. In the paper record, it is pretty easy to just take those three pieces of paper out whereas in an electronic environment, it is both easier to merge data and more difficult to remove incorrect data. So figuring out how to separate two records becomes equally challenging.
In terms measuring the accuracy of these algorithms is everyone using the same test?
I don't know that we've seen industry-wide agreement, which is part of why we put out the challenge. If we are going to talk about matching accuracy let's look at F score, let's look at percentages, let's look at recall, those are statistical benchmarks used for other types of analyses such as this and let's see if we can get everyone to agree that these are the ones we want to use.
Congress issued a clarification based on a letter 21 industry leaders wrote about the 1999 ban on national patient ID that relaxes the restrictions on ONC in terms of working with industry on patient identification? Has that affected what ONC is doing? Is the challenge a result of that change or was the challenge in the works before that happened.
The challenge was in the works before that and as I noted in the blog post, we have someone in a position called innovator-in-residence sponsored by the HIMSS organization. Two years ago they sponsored this position and we have been working on various areas related to patient matching, and that was sort of the focus of that position. One of the things we landed on was to do this algorithm challenge to do the benchmarking and get greater visibility and transparency in the market. The language provided by Congress is certainly helpful and provides stakeholders additional clarity around current expectations from Congress and are relative to our work.
When does the challenge begin and what are the deadlines?
The challenge is now open and will close on September 12th, 2017. During this time period, participants will be able to test their algorithms against our dataset. Participants will get 100 tries to submit their answers, get their scores and tweak their algorithms to see if it makes a difference in the next run-through. There is fierce competition at the top spots right now and you can check out the updates as part of the leaderboard on the challenge’s webpage.
Interested professionals can click The Patient Matching Algorithm Challenge to participate. The Challenge Submission Period Ends September 12, 2017.
No comments:
Post a Comment